Disclosure:
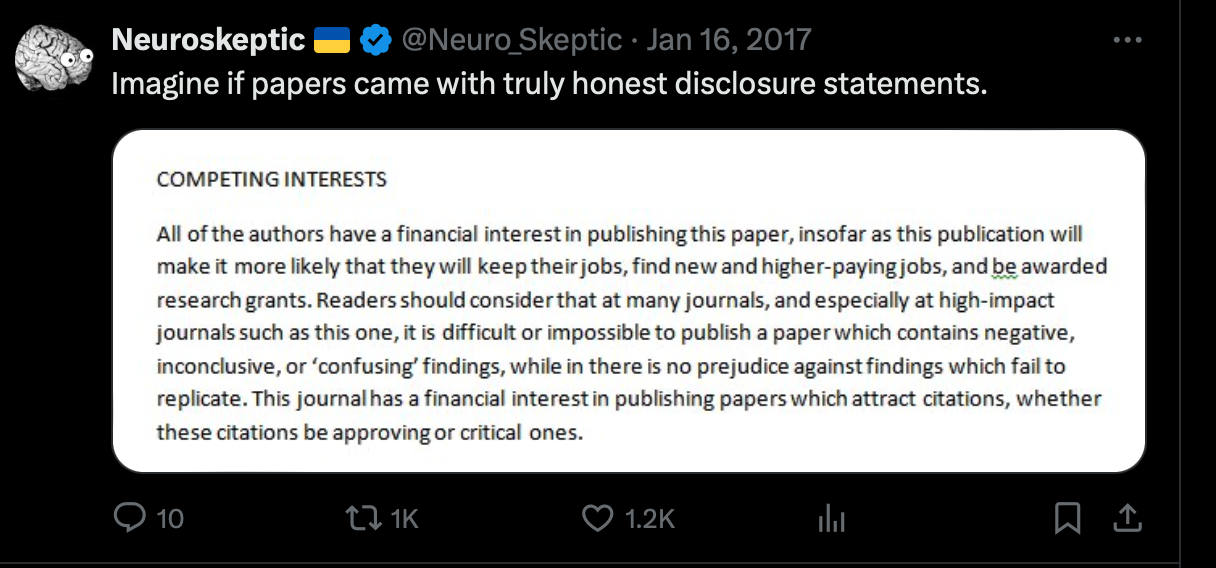
Disclosure:
The start of Cloudflare’s robots.txt
file: :-)
HTT: UL newsletter
Chuck Marohn of Strong Towns writes I’m Done With Twitter, but Not for the Reasons You Might Think with examples of how the platform undermined their efforts at constructive dialog.
Cyber-security is a broken-window fallacy, but there’s something delightful about this little bot tarpit:
The attacking bot reads the hidden prompt and often traverses the infinite tarpit looking for the good stuff. From Prompt Injection as a Defense Against LLM-drive Cyberattacks (two GMU authors!). HTT Unsupervised Learning (Daniel Miessler)
Timescale argues that special vector databases are the wrong idea because vectors are more like a special index derived from the data.
“Let the [general] database handle the complexity” of updating that “index” when the data changes. Because it will.
For some reason it’s hard to hold this in mind.
❝ | In MAGAworld, declarative statements ... serve as identity markers.... They are not for conveying Facts, Truth, Reality.... Whether ... Democrats have and deploy weather weapons could not be more irrelevant; what matters is that _this is the kind of thing we say about Democrats_ |
Would people who talk about weather weapons agree?
And was “Defund the police” similar?
Earlier AJ linked from a recent post. Insightful as usual. teachers at the margins – The Homebound Symphony
Bizarre editing glitch in a new Science article about possible image manipulation in Alzheimer’s studies:
Accordingalpha-synuclein and might to Prothena, the drug blocks the spread of toxic slow the progression of Parkinson’s movement disorders and dementia.
❝ | the peril of AI is the same as the promise of AI: it’s a thoughtlessness enabler. |
❝ | The difficulty of getting the answer was often a filter that ensured only those who understood the problem deeply would arrive at a solution. |
kozyrkov.medium.com/strawberrys-paradox, by Cassie Kozyrkov
An excellent article, though reading in full probably requires you join Medium.
Interesting abstract from Herzenstein et al suggests that replicable studies are transparent and confident relative to non-replicable ones.
Alas for itself, the abstract is timid. They “allude to the possibility that”.
Even if true, likely temporarily so. But I’ve requested the full text.
Daniel Lakens on why we won’t move beyond p<.05. Key: few really offered alternatives.
Part 2 of an excellent 2-part reflection on the APA special issue 5 years ago.
In-depth review of pending Metascience reforms at NIH by Stuart Buck at GoodScience. Excellent in-depth commentary.
This newsletter covers no fewer than four exciting metascience developments, with huge potential for improving science and medicine at NIH and elsewhere.
Including:
This is promising: you can run LLM inference and training on 13W of power. I’ve yet to read the research paper, but they found you don’t need matrix multiplication if you adopt ternary [-1, 0, 1] values.
Daniel Miessler suggests the Left is fueling Trump with a defeatist and anti-American narrative.
I started following Miessler for cyber-security. I like his generally centrist and wide-ranging take, and respect what he’s accomplished with hard work and self study. He’s onto something here.
The Shackles of Convenience, from Dan Miessler.
Found an old tab with a reminder that misinfo isn’t just on the Right.
I like being wrong?
❝ | The best way that a man could test his readiness to encounter the common variety of mankind would be to climb down a chimney into any house at random, and get on as well as possible with the people inside. And that is essentially what each one of us did on the day that he was born. |
Chesterton, Heretics -- On Certain Modern Writers and the Institution of the Family.
❝ | The modern man thinks that everything ought to be done for the sake of something else, and never for its own sake. |
In praise of idleness, by Bertrand Russel. (HTT Daniel Miessler.)
My neighbor’s astonishingly vivid azalea
Starting Harrow by Tamsyn Muir. 📚
Finished The Difficult Subject by (pen name) Molly Macallen, book 2 in the Maddy Shanks mystery series. 📚
Dr Shanks tries to resume her academic life while the trial from book one begins in Philadelphia. But her chair wants her to investigate the the surprising death of her predecessor.
Wim Vanderbauwhede estimates ChatGPT uses ~60x as much energy as Google search. (Geometric mean of other estimates, with analysis.)
“The first step is to draw a diagram. When you draw a diagram you’re loading it into your GPU. That is the key.” ~Casey Handmar on solving problems
The analogical case with humans would be where established authors, for example, sued new and upcoming authors for having learned their craft, in part, by reading the works of established authors.
I suggest the answer, however, is not twisting existing copyright law into performing new functions badly, but in writing new laws that directly address the new problems
~Kevin Korb, Generative AI Does Not Violate Copyright but Needs To